13. juni 2024
||
By Humzaa Imtiaz Ullah
Optimizing Chemical Process Design Through Advanced Data Analytics
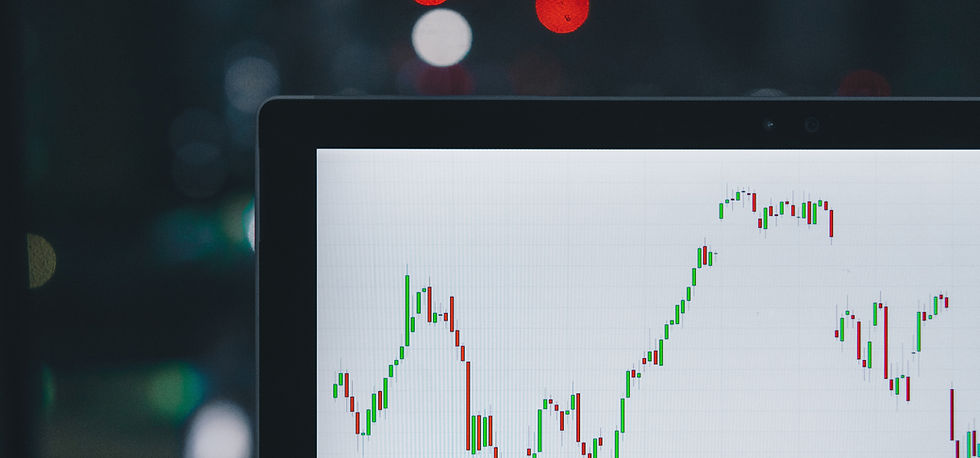
The chemical engineering landscape is experiencing a profound transformation, largely driven by the rise of advanced data analytics. Traditionally, chemical process design has depended on empirical methods, which often involve trial-and-error and heuristic approaches based on experience and established rules of thumb. While these methods have served the industry well for decades, they are increasingly being complemented—and in some cases, replaced—by data-driven techniques that offer a more precise, predictive, and adaptable framework for process design. This shift is enabling engineers to uncover insights that were previously hidden, optimize processes with unprecedented accuracy, and accelerate innovation in ways that were unimaginable just a few years ago.
The integration of advanced data analytics into chemical engineering brings a multitude of benefits. By leveraging vast amounts of process data, engineers can create highly detailed models that simulate chemical reactions and process flows more accurately than ever before. These models enable the optimization of operating conditions, the prediction of system behavior under various scenarios, and the identification of potential issues before they occur. Tools like machine learning, artificial intelligence, and big data analytics are now at the forefront of process design, allowing for the continuous improvement of processes in real-time, rather than relying on static models or past experiences alone. Moreover, this data-driven approach facilitates the design of more sustainable and energy-efficient processes, as it allows for the fine-tuning of resource use and the minimization of waste.
However, the integration of advanced data analytics into chemical process design is not without its challenges. The complexity of chemical processes, coupled with the vast amount of data generated, requires sophisticated tools and expertise to analyze and interpret the information effectively. Additionally, there are concerns about data quality, the need for robust data management systems, and the potential for resistance to change within organizations accustomed to traditional methods. Despite these challenges, the potential benefits—such as enhanced efficiency, increased innovation, and the ability to rapidly adapt to industry changes—make this an exciting and transformative time for chemical engineering. This article delves into how advanced data analytics is optimizing chemical process design, the cutting-edge tools and techniques involved, and the potential benefits and challenges that come with this integration, highlighting the promising future of a more data-driven industry.
The Role of Data Analytics in Chemical Process Design
Data analytics in chemical process design is a powerful tool that transforms vast amounts of raw data into actionable insights, driving informed decision-making across all stages of production. The process begins with the extraction, transformation, and analysis of both historical and real-time data, allowing engineers to optimize every facet of chemical production, from the selection of raw materials to the quality of the final product. By leveraging advanced data analytics, companies can gain a deeper understanding of their processes, predict future outcomes, and prescribe specific actions that enhance efficiency, reduce costs, and improve overall performance.
One of the foundational areas of data analytics is descriptive analytics, which focuses on analyzing historical data to identify trends and understand past performance. In the context of chemical engineering, descriptive analytics can reveal how different variables—such as temperature, pressure, or reactant concentrations—have influenced process outcomes over time. For instance, by examining past production data, an engineer might discover that small fluctuations in temperature led to significant variations in product quality. This insight can then be used to stabilize the process, ensuring more consistent outputs. Building on the insights gained from descriptive analytics, predictive analytics uses statistical models and machine learning algorithms to forecast future outcomes. This approach is particularly valuable in chemical process design for predicting equipment failures, optimizing maintenance schedules, and improving yield predictions. For example, predictive analytics might analyze data from sensors monitoring a reactor to anticipate when a component is likely to fail, allowing for maintenance to be scheduled before a breakdown occurs. This not only prevents costly downtime but also extends the lifespan of equipment and enhances overall plant reliability. Prescriptive analytics takes the next step by recommending specific actions based on predictive models. In chemical process design, this means providing engineers with optimal process parameters and configurations to achieve desired outcomes. For instance, if predictive analytics suggests that increasing the temperature slightly could improve yield without compromising product quality, prescriptive analytics would advise implementing this change. This approach can significantly enhance process efficiency, reduce waste, and improve the sustainability of chemical production.
By integrating these advanced data analytics techniques, chemical engineers can move from simply reacting to issues as they arise to proactively optimizing processes and preventing problems before they occur. This data-driven approach not only leads to more efficient and reliable chemical processes but also fosters innovation and helps companies stay competitive in a rapidly evolving industry.
Tools and Techniques
Several advanced tools and techniques are essential in applying data analytics to chemical process design, each contributing to more accurate modeling, comprehensive analysis, and real-time optimization of complex processes. These technologies empower engineers to move beyond traditional methods, embracing a data-driven approach that enhances efficiency, safety, and innovation across the industry.
Machine learning and artificial intelligence (AI) play a crucial role in this transformation. Algorithms such as neural networks, decision trees, and support vector machines are capable of modeling intricate chemical processes with remarkable accuracy. These models can analyze a vast array of input variables to predict outcomes, enabling more precise control and optimization of chemical processes. For instance, a neural network might be used to predict the yield of a chemical reaction based on factors like temperature, pressure, and reactant concentrations, allowing engineers to fine-tune these parameters for optimal performance. This level of precision not only improves efficiency but also reduces waste and energy consumption. Handling the massive volumes of data generated in chemical processes requires robust big data technologies. Platforms like Hadoop and Spark have revolutionized data processing by enabling the analysis of large datasets that were previously too cumbersome to manage. These tools allow engineers to perform a more comprehensive analysis of process data, uncovering patterns and correlations that might be missed with smaller datasets. For example, by analyzing years of production data from multiple plants, big data platforms can identify subtle trends that inform process improvements or reveal the underlying causes of inefficiencies.
In tandem with big data, simulation and modeling software such as Aspen Plus, MATLAB, and COMSOL Multiphysics provide a powerful environment for process simulation and optimization. These tools can integrate seamlessly with data analytics platforms, allowing engineers to simulate various process scenarios and optimize designs before implementation. For example, Aspen Plus might be used to model a distillation process, while data analytics tools help refine the model by comparing simulation results with real-world data. This integration leads to more accurate and reliable process designs, reducing the need for costly and time-consuming trial-and-error experimentation. The advent of the Internet of Things (IoT) and real-time analytics has further enhanced the capability of data-driven chemical process design. IoT devices, such as sensors embedded in equipment, continuously collect real-time data on process conditions. This data can be fed into real-time analytics platforms, which provide instant insights and feedback for process control. For instance, if a sensor detects an anomaly in reactor temperature, the real-time analytics system can immediately alert operators and suggest corrective actions, preventing potential issues before they escalate. This real-time feedback loop not only improves process safety but also enhances overall efficiency and reliability.
By leveraging these advanced tools and techniques, chemical engineers can optimize processes with greater accuracy, speed, and flexibility, driving the industry toward a more efficient, innovative, and sustainable future.
Case Studies and Applications
Several case studies exemplify the successful application of advanced data analytics in chemical process design, demonstrating how these techniques can lead to significant improvements in efficiency, safety, and cost-effectiveness.
Catalyst Optimization is a prime example where machine learning algorithms have made a profound impact. In a petrochemical plant, historical data on catalyst performance was analyzed using sophisticated machine learning models. These models helped identify the optimal conditions for maximizing catalyst activity, such as temperature and reactant concentrations. As a result, the plant achieved increased yield and reduced operating costs. For instance, by optimizing catalyst usage, the plant could increase the production of valuable chemicals while minimizing the need for costly catalyst replacements and maintenance.
Process Safety Management has also benefited from data analytics, particularly through data mining techniques applied to historical incident data. By analyzing past safety breaches, patterns and common causes were identified, leading to the development of more effective safety protocols. For example, data mining might reveal that a series of incidents were linked to specific equipment malfunctions or procedural lapses. With these insights, the plant could implement targeted interventions, such as enhanced training programs or upgraded safety systems, significantly reducing the likelihood of future incidents and improving overall safety standards.
In the realm of Energy Efficiency, predictive analytics have proven invaluable. A chemical manufacturing facility utilized predictive models to analyze energy consumption patterns and pinpoint areas where energy savings could be realized. By adjusting process parameters based on these insights, such as optimizing reaction conditions or improving insulation, the facility achieved a 15% reduction in energy consumption. This not only led to substantial cost savings but also contributed to the facility's sustainability goals by lowering its carbon footprint.
These case studies illustrate how advanced data analytics can drive transformative improvements in chemical process design, from optimizing catalysts and enhancing safety to achieving greater energy efficiency. By leveraging these powerful tools, industries can unlock new levels of performance and sustainability, paving the way for a more efficient and environmentally responsible future.
Challenges and Future Directions
While the integration of advanced data analytics into chemical process design offers substantial benefits, several challenges need to be addressed to fully realize its potential.
Data Quality and Integration is a significant challenge. Ensuring the accuracy and reliability of data is crucial for developing effective models and making informed decisions. In chemical process design, data often comes from various sources, including sensors, historical records, and simulations. Integrating this disparate data into a cohesive system can be complex and time-consuming. For instance, if data from different sensors have varying levels of accuracy or are recorded in incompatible formats, it can lead to flawed models and suboptimal process optimizations. Ensuring high-quality data and seamless integration is essential for accurate analysis and reliable outcomes. At the same time, the skill gap in the industry is another notable challenge. The field of data analytics requires a different set of skills compared to traditional chemical engineering. Chemical engineers often need to be proficient in data science, machine learning, and statistical analysis to effectively apply these techniques. This skill gap highlights the need for cross-disciplinary education and training programs that can equip engineers with the necessary expertise. For example, universities and industry organizations are increasingly offering specialized courses and certifications in data analytics for chemical engineers, helping to bridge this gap and prepare professionals for the evolving demands of the field.
Implementation costs present a further obstacle. The initial investment required for data analytics infrastructure, including advanced software, hardware, and skilled personnel, can be substantial. These costs can be a barrier for some organizations, particularly smaller companies with limited budgets. However, despite the high initial costs, the long-term benefits often outweigh the investment. For instance, the improved efficiency, reduced operational costs, and enhanced safety resulting from advanced data analytics can lead to significant financial gains over time. Organizations that invest in these technologies are likely to see a return on their investment through improved process performance and competitive advantage.
Addressing these challenges is crucial for the successful integration of advanced data analytics into chemical process design. By focusing on data quality, bridging the skill gap, and evaluating the long-term value of investments, companies can effectively harness the power of data analytics to drive innovation and achieve greater efficiency in their processes.
Looking ahead, the landscape of chemical process design is poised for further transformation through the integration of quantum computing, enhanced machine learning algorithms, and more sophisticated IoT devices. Quantum computing holds the promise of solving complex optimization problems that are currently beyond the reach of classical computers, potentially enabling unprecedented precision in process design and simulation. Enhanced machine learning algorithms will improve the ability to predict and control process variables with greater accuracy, learning from vast amounts of data to refine models and make real-time adjustments. Additionally, advancements in IoT technology will enable more detailed and accurate monitoring of chemical processes, providing a wealth of real-time data that can be used to optimize operations and enhance safety. These innovations will not only increase efficiency but also push the boundaries of what is possible in chemical engineering, paving the way for breakthroughs that could revolutionize the industry.
As advanced data analytics continues to evolve, it will play an increasingly central role in transforming chemical process design. The integration of machine learning, big data technologies, and real-time analytics is set to offer unprecedented opportunities for optimization and innovation. Chemical engineers will be able to design processes that are more efficient, cost-effective, and environmentally sustainable, driven by data that provides deeper insights and more precise control. The ability to harness these technologies will lead to safer operations, reduced waste, and enhanced overall performance. As the industry embraces these advancements, the future of chemical engineering promises to be both dynamic and promising, with data analytics at the core of this exciting evolution.